Insurance Data May Predict ALS, Research Shows
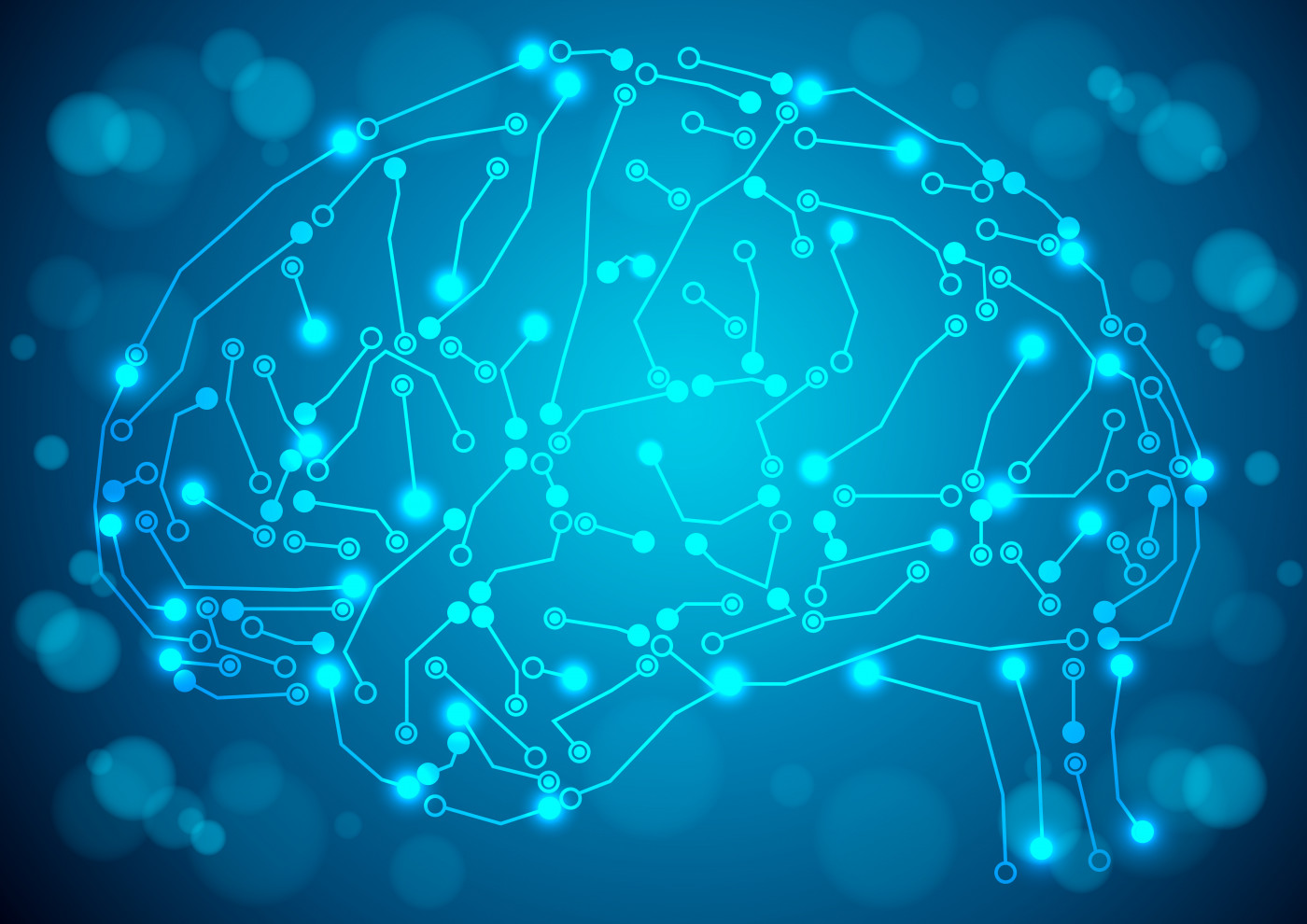
A machine-learning model based on insurance information from amyotrophic lateral sclerosis (ALS) patients may be able to speed diagnosis of the disease in others, research suggests.
The findings were presented at the 30th International Symposium on ALS/MND, in the presentation “Machine learning model using insurance claims data to help predict future ALS diagnosis” (abstract EPI-10). The research was supported by Mitsubishi Tanabe Pharma America (MTPA).
It often takes a year or more after symptoms begin for people with ALS to be diagnosed correctly, a process that may require visiting several different specialists. This lengthy delay can be stressful and prevents patients from beginning treatment that could delay disease progression.
Researchers wondered if ALS could be predicted based on a person’s history of insurance claims — the theory being that insurance claims represent patterns of health history that might be similar among people with ALS.
To test this, they used machine learning, a technique that involves feeding data into a computer and developing algorithms for a specified task. Insurance claim data is particularly amenable to machine learning because there is so much of it, meaning there are many data points with which to construct algorithms. While a human can’t feasibly make sense of all that data, computers can.
In this case, the researchers fed in insurance data for 5,047 ALS patients and 50,470 people without ALS (the controls) matched to patients by their demographics, such as age, gender, and where they live. Then, using these data, the computer developed algorithms to separate those with ALS from those without.
The researchers then tested the algorithm using a separate dataset, with data for 1,227 patients and 11,472,450 controls.
The model was able to identify patients and controls with an accuracy of 74.5%. Depending on the score used as threshold, the model reached a positive predictive value (PPV) — the percentage of people identified as positive who actually have the disease — of 14.3%.
The PPV “greatly exceeded the actual prevalence of ALS” in the overall population, the researchers wrote. In other words, based on this data and from a purely statistical standpoint, the model likely would be able to identify nearly all people with ALS, which the researchers suggested could shorten time to diagnosis by as much as a year in some cases. They said the model could be improved with the inclusion of other types of data — laboratory results, for example.
The findings, along with others MTPA presented at the symposium, will “provide important insights as we work to increase understanding of ALS and the patient journey,” Atsushi Fujimoto, the company’s president, said in a press release.