Machine Learning May Help Predict ALS Progression
The longer the delay in diagnosis, the worse the outcome, study also finds
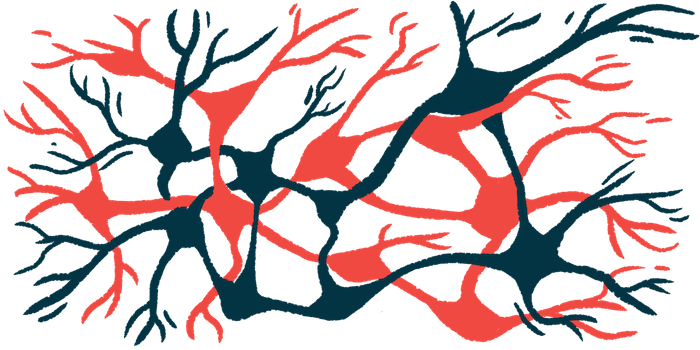
Deep machine-learning models using neural networks — computer algorithms inspired in the biological networks of the human brain —may help to predict the course of amyotrophic lateral sclerosis (ALS), a new study reports.
The models also consistently showed that a longer delay between disease onset and diagnosis is a powerful predictor of worse long-term outcomes, emphasizing the importance of early diagnosis.
The study, “Deep learning methods to predict amyotrophic lateral sclerosis disease progression,” was published in Scientific Reports.
ALS is a notoriously hard-to-predict disease — the rate of progression can vary substantially from person to person, and uncertainty about the prognosis can make it harder for patients and their families to plan for the future.
Machine learning is a form of analysis that basically involves feeding a computer a bunch of data, along with a set of algorithms the computer uses to “understand” the data. This allows the computer to “learn” how to make sense of the data according to the prescribed mathematical rules. A burgeoning field of research is exploring how machine-learning models might be applied to predict outcomes in ALS and other diseases.
For this study, scientists in Italy created machine-learning algorithms called “neural networks” aiming to predict ALS progression. A neural network machine-learning algorithm uses a series of procedures designed to mimic how information is processed by networks of neurons (nerve cells) in the brain.
The team specifically crafted and tested three different types of neural network structures, referred to as “feed-forward,” “convolutional,” and “recurrent.”
To develop and test the models, the researchers used data from the Pooled Resource Open-Access ALS Clinical Trials (PRO-ACT) repository, a database that collects long-term outcomes and clinical information from ALS patients who participated in clinical trials.
PRO-ACT “provides the largest publicly available dataset of merged ALS clinical trials” with “longitudinal data for more than ten thousand patients,” the researchers wrote. However, they also noted that the dataset “is not representative of a general ALS distribution,” as patients in the dataset are generally younger and closer to disease onset.
Using data from more than 250 different demographic and clinical variables, the neural networks were trained to predict outcomes on the ALS Functional Rating Scale (ALSFRS), a standardized measure of disease progression. The neural network algorithms were compared against two previously established machine-learning algorithms called Random Forest Regressor (RF) and Bayesian Additive Regression Trees (BART).
Statistical tests suggested that all of these models had similar performance, with some relative pros and cons for each. Compared to RF and BART, the neural network models generally had smaller ranges of error, but also slightly less accuracy overall compared to BART. Among the neural networks, the “convolutional” model generally showed the best performance.
“While deep learning [neural network] models performed comparably to state-of-the-art models, they did not provide a decisive advantage,” the researchers wrote, noting that the neural networks showed “a slight advantage” in some statistical tests, but “a slight disadvantage” in others.
Most predictive factor
In all of these models, the single factor that was most predictive of overall outcomes was the time between the onset of ALS symptoms and the time when the disease was diagnosed.
The researchers also tested whether the models could predict survival outcomes. Results generally showed weak correlations. In other words, the models were not very accurate for predicting survival outcomes in individual patients. But results showed the models could accurately divide patients into “fast progressors” and “medium-slow progressors”, with consistently better long-term survival rates in the slower progression group.
“Despite the limits observed in the model performance, we showed that the predicted ALSFRS slope is able to distinguish fast progressors with worse survival from medium-slow progressors and is thus predictive of concrete clinical events in the patient history,” the researchers concluded.