Machine Algorithm Ably Predicts Changes in Lung Function in ALS Patients in Clinical Trial
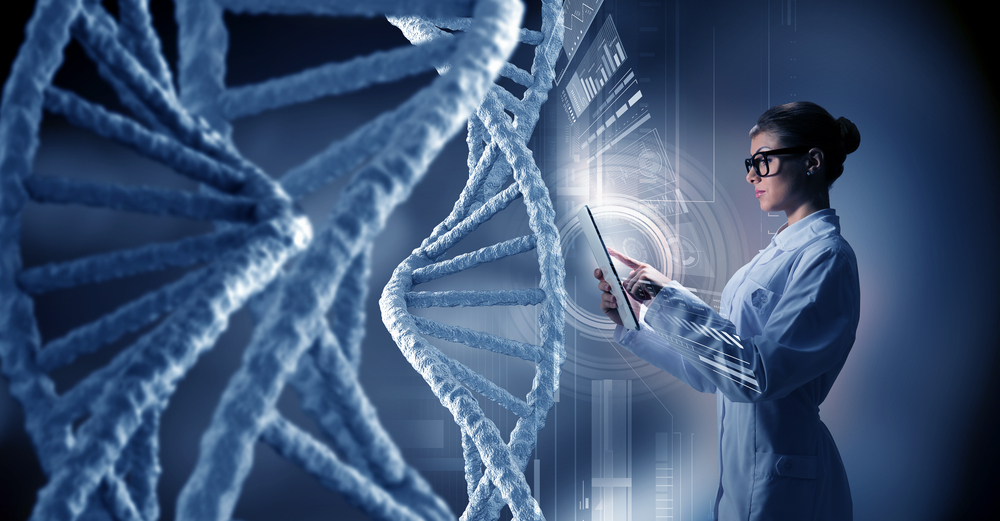
Origent Data Sciences and Cytokinetics reported results from the first part of a research partnership to further refine and validate an Origent’s computer model to predict amyotrophic lateral sclerosis (ALS) outcomes and disease progression. The effort’s ultimate goal is to develop models that can replace control arms, and the need for placebo groups, in ALS clinical trials.
The computer model is using data from Cytokinetics’ late-phase human clinical trials of tirasemtiv, a skeletal muscle troponin activator, in ALS patients. The results, the first prospective validation of predictive models in a clinical trial setting, were recently presented at the 27th International Symposium on ALS/MND, held in Dublin.
Data revealed that the Gradient Boosting Machine (GBM) algorithm was the best model for predicting slow vital capacity (SVC, a measure of lung function) subsequent to baseline. Using this machine learning technique, records of forced vital capacity (FVC) also predicted SVC scores of patients in the studies.
Origent models, predicting both function and survival of patients with ALS, have been previously validated using internal and retrospective external datasets.
“We are pleased to have completed this first-ever step toward using machine learning models for prospectively predicting ALS outcomes and disease progression in late-phase human clinical trials, and look forward to continuing this important collaboration with Cytokinetics,” Dave Ennist, chief science officer for Origent, said in a press release.
“These data bring us one step closer to prospectively validating the Origent predictive model using datasets from BENEFIT-ALS and VITALITY-ALS,” said Jinsy Andrews, MD, director of Neuromuscular Clinical Trials at Columbia University and the medical monitor for the VITALITY study. “We hope that predictive algorithms arising from this research collaboration may ultimately accelerate clinical trials in patients with ALS by using virtual control arms.”
In the first part of this project, to predict SVC at set times, the researchers created a computer model that utilizes baseline patient characteristics retrieved from PRO-ACT (Pooled Resource Open-Access ALS Clinical Trials), an ALS clinical trials dataset that contains over 8,500 patient records from multiple completed trials. For this prediction model, the team used data from the Phase 2 BENEFIT-ALS (NCT01709149) and Phase 3 VITALITY-ALS (NCT02496767), two large studies that evaluated the efficacy and safety of tirasemtiv in improving lung function in ALS patients.
Using PRO-ACT, the researchers compared three predictions models, and the GBM algorithm was the best in predicting SVC. They determined a total of 22 baseline patients’ characteristics, including FVC, that could be used to train a model that predicts SVC at subsequent times.
The researchers also demonstrated that baseline FVC was highly correlated with SVC, and that FVC at baseline was the most important predictor of vital capacity at subsequent times in ALS patients.
ALS is a disabling and progressive neurodegenerative disease that affects respiratory muscles and pulmonary function. Vital capacity, slow vital capacity (SVC) or a forced vital capacity (FVC) are the most critical pulmonary measurements to assess disease progression. Tirasemtiv, a novel skeletal muscle activator, is designed to trigger the muscle troponin complex, increasing its sensitivity to calcium, a key regulator of muscle contraction.