University of Michigan Develops New Model for Predicting ALS Patients’ Survival
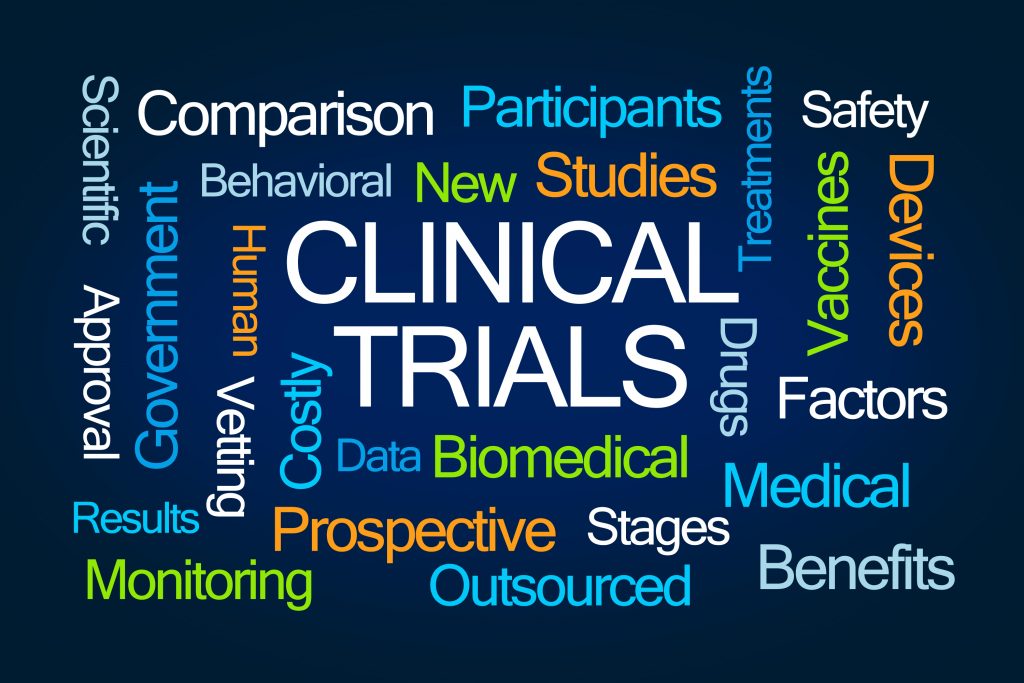
A new model for predicting ALS patients’ survival looks promising, University of Michigan researchers report.
In fact, it want a competition for models that can forecast survival.
A combination of patients’ ability to walk, breathe and eat, plus their scores on a physical functioning scale and a lung function measure are strong predictors of survival, the team said. The physical functioning scale is ALSFRS, and the lung function measure is forced vital capacity, or how much air a person can exhale after taking a deep breath.
The research, “Complete hazard ranking to analyze right-censored data: An ALS survival study,” appeared in the journal PLOS Computational Biology.
Survival analysis is based on factors that can collectively estimate patients’ survival time. It is crucial to evaluating the effectiveness of a new therapy in clinical trials.
The most commonly used survival prediction model — the Cox proportional hazards — can lead to wrong forecasts if incorrectly applied. So researchers have been developing variations to this model and other statistical tools.
Recent developments in machine learning have shown promise of increasing the accuracy of survival analysis. Machine learning is an artificial intelligence application that allows computers to learn by themselves to perform tasks better and better.
The University of Michigan scientists developed a new hazard ranking algorithm, or procedure that computers can use to solve a mathematical problem. Called GuanRank, it transforms patients’ data into hazard ranks.
To test the model, the team used information from the PRO-ACT database of ALS clinical trials. They validated it in the DREAM Amyotrophic Lateral Sclerosis Stratification Challenge.
GuanRank came in first in the competition, predicting patients’ survival more accurately than conventional models.
The components of the GuanRank model that the researchers identified for ALS survival “may suggest that optimizing respiratory treatments can improve ALS disease management,” the team wrote.
In contrast with previous studies, patients’ age at disease onset and their uric acid levels were not found to be predictive of survival. Significant differences in the age of the patients who were used to develop the model may be why age didn’t become a component of it, the researchers said.
Taking the ALS medication Rilutek (riluzole) was also not a strong predictor of survival. This may be because the patient information did not distinguish long-term from short-term users of the therapy, and because many patients were healthier than the typical ALS population.
Researchers plan to test the GuanRank model in other datasets, including those for different diseases.
Overall, “the method demonstrated its state-of-the-art predictive power in ALS survival ranking,” the team concluded.