AI Finds New Potential Targets for ALS Treatment in Patient Data
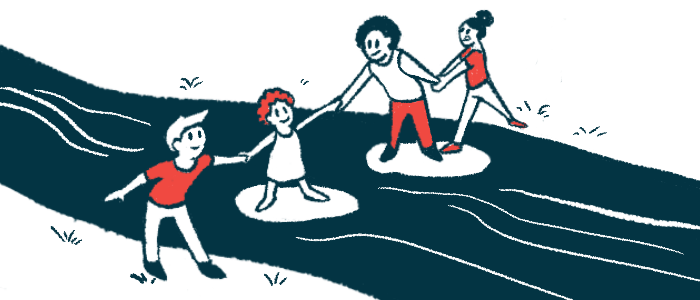
PandaOmics, an artificial intelligence (AI) platform that looks at vast amounts of data to find “druggable” molecules, has identified more than two dozen new targets with therapeutic potential for amyotrophic lateral sclerosis (ALS), according to a recent study.
Target discovery is a first step in the path to therapeutic development. While it’s too early to know if any of the newly found targets will lead to an effective treatment, the study taps into AI’s potential as a way to find better molecular targets much faster than current approaches.
“AI speeds up the target discovery process, and opens up new opportunities for therapeutic interventions,” the researchers wrote.
The study, “Identification of therapeutic targets for amyotrophic lateral sclerosis using PandaOmics – an AI-enabled biological target discovery platform,” was published in Frontiers in Aging Neuroscience.
The study was part of a collaboration between Insilico Medicine, which developed the AI discovery platform, and investigators at a number of research institutions and Answer ALS, the largest ALS research program that stores information from hundreds of patients and healthy people.
“The results of this collaborative research effort show what is possible when we bring together human expertise with AI tools to discover new targets for diseases where there is a high unmet need,” Alex Zhavoronkov, PhD, founder and CEO of Insilico, said in a company press release. “This is only the beginning.”
ALS occurs when motor neurons (the nerve cells in the brain and spinal cord that control movement) gradually become damaged and die over time. As a result, the muscles begin to waste and become too weak to work as they should. In some patients, the disease is linked to known genetic mutations. In most cases, however, there is no clue about what causes the disease, which can complicate treatment.
The researchers drew on data from public databases and Answer ALS to find new genes that could be relevant to the disease, which could identify new targets with therapeutic potential.
These included publicly available brain and spinal cord samples from 237 patients with ALS and 91 healthy people, as well as patient-derived motor neurons from 135 patients and 31 healthy people included in Answer ALS.
To handle such a large amount of data, the researchers relied on PandaOmics whose platform uses a set of algorithms to run through a large pool of data and rank a list of candidate targets based on relevant measures.
In the study, that list retrieved 28 new targets. Of those, 17 were picked based on how likely they were to be studied as part of a funded research project and how relevant they were considered by someone working in the field. These were deemed high-confidence targets.
The remaining 11 targets were selected based only on biological measures, such as their accessibility by small molecules, novelty, and potential safety profile.
“We are truly excited to see the Answer ALS data being used to identify possible ALS disease-causing pathways and candidate drugs,” Jeffrey D. Rothstein MD, PhD, who directs the Robert Packard Center for ALS Research and Answer ALS, said. “The work by Insilico is exactly how this unprecedented program was envisioned to help change the course of ALS.”
To validate the identified targets, the team used a fruit fly model carrying a repeat expansion in the C9orf72 gene, the most common genetic cause of ALS. Of the 28 candidate genes, 26 had a corresponding gene in flies and were examined for their effects in ALS-like symptoms.
If left untreated, these flies experience alterations in eye shape and function consistent with neurodegeneration. But inhibiting 18 of the targets rescued these alterations. The effect was opposite for one target (RPS6KB1), with its inhibition leading to even more severe symptoms.
“It is impressive that around 70% (18 out of 28) targets identified by AI were validated in a preclinical animal model,” Feng Ren, PhD, co-CEO and chief scientific officer of Insilico, said.
Of these, eight (KCNB2, KCNS3, ADRA2B, NR3C1, P2RY14, PPP3CB, PTPRC, and RARA) had never been reported.
All the targets were found to participate in processes that may contribute to developing ALS. Some examples are processes that control immune response and cell death.
“It is exciting to see the power of AI to help understand ALS biology,” Merit Cudkowicz, MD, who co-led the study, said. Cudkowicz is chief of neurology and director of the Healey & AMG Center for ALS at Mass General Hospital and Harvard Medical School.
Ren said Insilico was working with its collaborators to bring some targets toward clinical trials for ALS. “At the same time, we are also further expanding the utilization of PandaOmics to discover novel targets for other disease areas including oncology, immunology, and fibrosis,” he said.