Researchers develop algorithm to predict ALS survival
The model estimates the time until patients reach respiratory failure
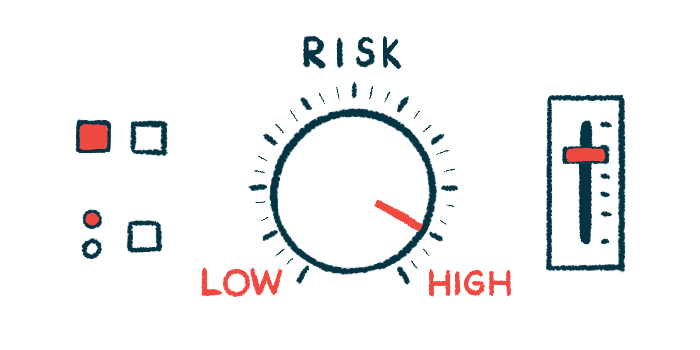
Researchers have developed an algorithm to generate personalized survival predictions for people with amyotrophic lateral sclerosis (ALS) based on clinical and MRI features. The scientists believe the algorithm could help patients and doctors make better treatment and care plans.
“The disease progression varies greatly for ALS patients, so it is important to consider their condition when planning future treatments. Our learned model considers the personal descriptions of the patient when giving survival curve predictions,” the researchers wrote in “Accurate personalized survival prediction for amyotrophic lateral sclerosis patients.” The study was published in Scientific Reports.
ALS is a rapidly progressing neurodegenerative disease characterized by muscle weakness and wasting that affects the muscles needed for moving, speaking, and breathing. The most common cause of death is respiratory failure.
While therapies can help slow the disease’s progression, most patients survive an average of about 2-5 years after the onset of symptoms. Survival time can range from a few months to more than 10 years for some patients, however, the researchers said.
“This range makes it impossible for clinicians to effectively counsel patients on advance care planning, or to select patients with specific survival characteristics for drug trials,” the researchers wrote.
Better ways of predicting survival time in ALS are needed to guide clinical care. While some models have been developed, they come with limitations. Most approaches don’t estimate exactly how long a person will live, but instead predict the probability of survival after a certain amount of time.
Predicting ALS survival time
The researchers worked to develop a personalized prediction model to estimate the time until a patient will reach respiratory failure, an indicator of survival, based on clinical and MRI features.
They used machine learning, wherein an algorithm learns to predict an outcome — in this case, survival — by examining patterns across a large amount of patient data. Here, the model learned by analyzing data from 172 patients in the Canadian ALS Neuroimaging Consortium, a long-term MRI-based study. The patients, who had an average age of 59.7, had had symptoms for an average of a little more than two years.
The algorithm took into account 114 clinical features, such as disability, cognitive function, disease duration, age, and sex, among others, along with 68 MRI features related to the thickness of the brain’s cortex, a measure of brain tissue wasting.
Data were included from the time of enrollment in the study until the time of death or the patient’s last follow-up. Overall, 72% of patients (95 of 172) had a recorded composite respiratory failure event, defined as death, used noninvasive ventilation for more than 23 hours per day, or needed a breathing tube placed.
From this information, the so-called SuperLearner algorithm selected an individual survival distribution (ISD) for each evaluated patient. The ISD provides the probability of survival at multiple points in time.
“Our learned model gives a personalized prediction specific to this patient rather than only telling the patient the average survival time of all ALS patients,” the researchers said.
The algorithm was found to have an accuracy of 77% for one-year survival, 57% for two years, and 85% for three years. As an example, the researchers presented the predicted survival curve for Patient A, where it was estimated based on the algorithm the patient would survive 40.4 months (a little less than 3.5 years). The patient died after 42.8 months. Similarly, a patient with a predicted survival of 8.3 months died after 1.5 months.
A strength of the machine learning model is that it takes into account and learns from patients who reached respiratory failure (called uncensored data) as well as those where data collection was stopped (censored) at a follow-up time before that outcome was reached.
“The simplifed approach of just testing on the uncensored instances can lead to biased results because patients whose events happen early are less likely to be censored (e.g., a patient who died on day 1 is less likely to be censored than a patient who died on day 1,000),” said the researchers, who noted the study “provides a way to predict the time until composite respiratory failure, as this will help patients make decisions about future treatment or care.”