Researchers Develop New Tool to Aid in Rare Disease Gene Discovery
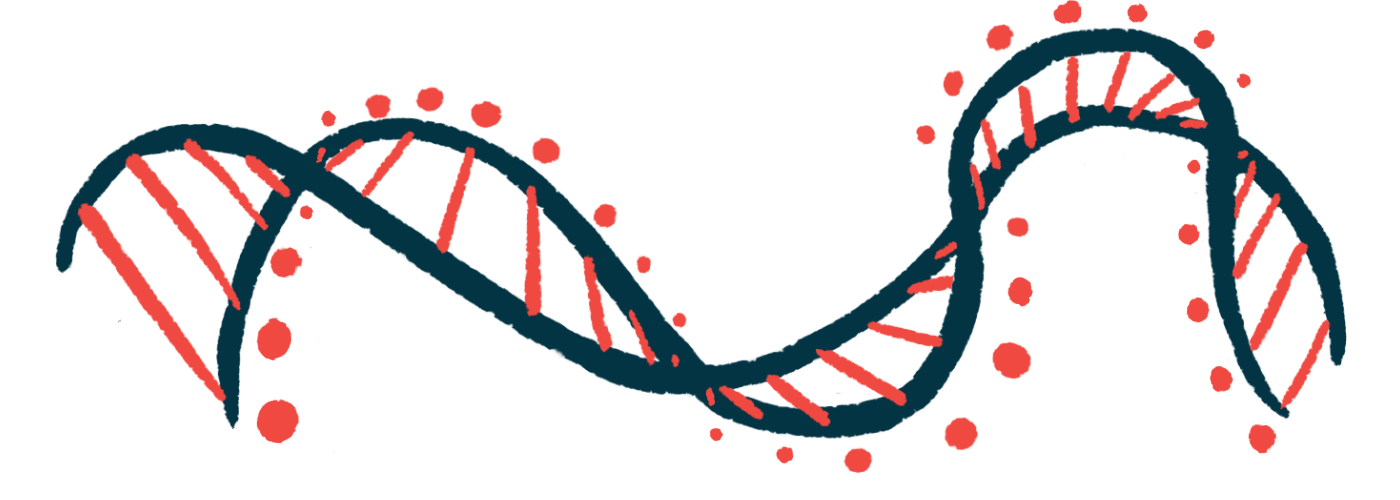
A research team has developed a new way to search for genes and gene mutations that may contribute to the development of rare diseases, bypassing some of the challenges typically associated with genetic analyses.
For diseases like amyotrophic lateral sclerosis (ALS) in which genetic factors are thought to contribute, this new approach could help scientists more reliably discover new genetic underpinnings and disease mechanisms.
In ALS, and other diseases like pediatric brain cancer, an estimated “20% of the patients can be explained by [genetic] predisposition to the disease,” Gang Wu, PhD, the director of the St. Jude Center for Applied Bioinformatics and the study’s senior author, said in a press release.
“Our tools will help find the remaining unexplained heritability that can be contributing to those diseases,” Wu added.
The study, “A rare variant analysis framework using public genotype summary counts to prioritize disease-predisposition genes,” was published in Nature Communications.Â
Genetic analyses help scientists to better understand rare disease mechanisms and offer insights into diagnosis and treatment. Often, disease-associated genes are identified by comparing genetic sequencing data from a small group of rare disease patients with those from age-matched healthy people included in a large public database.
But finding genetic variants that truly cause rare diseases can be extremely difficult. Usually, there are few patients with the rare disease, and it is also difficult to find a group of healthy, age-matched participants who are similar enough to the patients to make a meaningful comparison.
Ultimately, this means that there are not enough participants and not enough consistency in the available data to allow any findings to reach statistical significance. In other words, the studies are limited in their ability to detect definitive relationships between a gene or mutation and a disease of interest.
“This is all a numbers game,” Wu said. “Traditionally, if you have a small cohort study of 20 to 50 unrelated individuals with a very rare disease, you have almost no way to find a novel gene variant that reaches statistical significance in its contribution to the disease without prior knowledge of candidate genes.
“Now we have an approach that can potentially help find novel disease predisposition genes,” Wu added.
In this new approach, Wu and colleagues created a tool called CoCoRV, or the consistent summary counts-based rare variant burden test. In brief, the test compares genetic data from rare disease patients with summary data that already exists in large public databases to identify potentially disease-causing genetic mutations, or variants.
Since the data from the two groups come from different sources, CoCoRV also utilizes a series of standardized quality control filters to ensure that any confounding factors that might obscure the findings are removed. Only “high-quality variants” are used in the analysis, the team wrote.
This pipeline essentially creates a control group that is a well-matched comparison for the disease group.
“When you have a large amount of data, you can use this knowledge to derive rules that systematically categorize what is a true signal versus which are bad quality in other datasets. We built that experience into a tool that would be helpful for others to use,” said Wenan Chen, PhD, the study’s first author.
“Users can therefore confidently scan for potential pathogenic [disease-causing] variants or try to identify risk genes for a rare disease,” Chen said.
To put CoCoRV to the test, the team used it to search for genetic variants in a dataset from an ALS study compared with healthy control data. The analysis included 3,093 ALS cases and 8,186 healthy controls, all of whom were Caucasian.
The analysis revealed that known ALS genes SOD1, NEK1, and TBK1 appeared to be among the top genes associated with ALS in the study group, validating the approach. The analysis was also able to identify and eliminate “false positive” results, the researchers said.
The researchers also validated their approach in an analysis of genetic data from cancer patients.
While the tool allows the prioritization of genes that might contribute to disease, “we caution that CoCoRV should be used as a prioritization tool and not a statistical validation tool,” the researchers wrote.
“Once interesting genes are identified, either a strict full-genotype-based association test or lab-based functional studies are needed to validate the findings,” they added. Genotype refers to the genetic composition of an organism.
The team has made the tool freely available to researchers studying rare diseases.